Master Theses
Please submit your topic of choice by filling out the form below:
Simulating Structural Dynamics with Graph Neural Networks

Supervisors: Gregory Duthé (), Kostas Vlachas (), Prof. Dr. Eleni Chatzi
Content
Graph Neural Networks (GNNs) have recently emerged as a powerful way to learn the dynamics of a physical process. Using standard meshing techniques, it is easy to decompose a physical problem, such as the dynamical simulation of a structure into a discrete graph, which a GNN can then operate on. In this thesis, we investigate the use of GNNs as alternative structural dynamics simulators. We will explore operations on graphs (incl. message passing) and their equivalence to sub-structuring and condensation schemes. It will also be important to include as many physics-based inductive biases as possible.
The student(s) will have the following objectives:
- Review the existing methods and literature
- Become familiar with the PyTorch Geometric framework
- Implement a first prototype, based on existing work from the literature
- Formulate methods for sub-structuring/condensation schemes which exploit the expressivity of GNNs
- Demonstrate the method on a simple case study
Suggested Courses: 101-0157-01L Structural Dynamics and Vibration Problems
Suggested Competencies: Python, PyTorch (or equivalent ML framework)


Digital twins – a small-scale demonstration
Supervisors: Dr. Yves Reuland (), Dr. Panagiotis Martakis, Dr. Larisa Garcia-Ramonda Estevez, Prof. Dr. Eleni Chatzi
Content
Many existing structures are nearing or passed already the service life they were designed for. With increasing traffic loads, the evolution of design codes, and material ageing engineers are tasked with assisting challenging decisions related to extending the lifetime of existing structures. The evaluation of existing bridges relies on computational models. Such representations of real structures, often termed as digital twins, should reflect real behavior as closely as possible and therefore, ideally involves monitoring data for calibration. Unvalidated modelling assumptions may lead to unrealistic evaluations, due to various sources of uncertainty and systematic errors:
• When real safety is lower than the model prediction, the assessment is insufficient and possibly unsafe.
• In case the real safety is higher, the assessment is overconservative and translates to unnecessary and expensive retrofit activities
With the use of a simple experimental structure, the entire chain of structural health monitoring, from measuring, through model calibration, until behavior prognosis will be addressed and validated. Such a holistic approach introduces the student into the necessary steps to develop a robust and accurate digital twin of an existing structure. Master thesis on monitoring-driven digital twinning of structures allows students to increase and deepen their knowledge of key aspects of structural dynamics, structural health monitoring, numerical modelling, uncertainties, and assessment of existing structures; through following the following steps:
- Design a monitoring campaign for a simple experimental specimen
- Develop numerical models of the experimental specimen
- Calibrate the numerical model based on the vibration monitoring of the specimen
- Estimate the uncertainties pertaining to the measurement and the model
- Study the influence of the amount and location of sensors on the model calibration
- Predict the structural behavior under new loading scenarios
Suggested Courses: Seismic Design


Finite-Element Updating Using Physics-Informed Neural Networks
Supervisors: Dr. Marcus Haywood-Alexander Marcus (), Prof. Dr. Eleni Chatzi
Content
Many high-value structural systems (such as bridges or wind turbines) monitor vibration data as a means of detecting faults within the structure or system. Modal characteristics of such systems can act as direct indicators of changes in local physics properties, and finite-element-model updating provides an approach to identify these properties on a finer resolution. With the explosion of data availability and data-driven machine-learning techniques, these have been used to drive such complex models. However, they require a large amount of data to adequately capture the model of modal characteristics to physical properties. Physics-informed are a popular new class of physics-enhanced machine learning algorithms, which utilise known physics in the form of ordinary/partial differential equations to promote the machine learning algorithm towards physically-informed solutions. By including the physical-properties as learnable parameters in training, PINNs can be used in a system-identification task.
The aim of this thesis would be to study the application of combining finite-element models with physics-informed neural networks to estimate local physical properties of a dynamic structure. In this thesis, the student will be expected to:
- Review the current state-of-the-art in literature
- Become familiar with physics-informed neural networks, and apply them to simple problems
- Develop a method for combining finite element models and physics-informed neural networks (FE-PINNs)
- Study the use of FE-PINNs in the context of novelty detection via model-updating, applying them to data from simulated or experimental data
Suggested Courses: Structural Identification and Health Monitoring, course on machine learning methods
Suggested Competencies: Python/MATLAB

Improving the design of rotating parts of wind turbine through metamaterial technology

Supervisors: Dr. Vasilis Dertimanis (), Prof. Dr. Eleni Chatzi
Industry Partners: Dr. Luca Sangiuliano, Phononic Vibes
Content
In the context of novel lightweight materials for noise and vibration reduction, Phononic Vibes Srl (https://phononicvibes.com/) is researching, designing and producing metamaterial technology. Metamaterials are often periodic structures, consisting of a host structure with embedded or added resonant structures, leading to superior vibro-acoustic attenuation performance in some tunable frequency ranges, called stop bands. In the following videos, the metamaterial concept is applied structure-borne (https://www.youtube.com/watch?v=DUee93HcPVQ&t) and airborne noise problems.
The resonant structures often have some small features that control their tuned frequency and the dynamic behavior of the entire metamaterial system. Once these resonant structures are applied to rotating systems, the stop band behaviour can be modulated according to the rotation speed to the entire system thanks to the presence of inertial forces (ref. 1). This interesting phenomenon can give rise to new methods to control the dynamic behavior of rotating systems and to reduce the unwanted dynamics.
Rotating parts of wind-turbines are often subjected to extreme dynamic conditions. To ensure the long service life and optimal design of rotating parts of wind-turbines, thus preventing failures and expensive maintenances, the dynamic behaviour of these parts must be thoroughly designed and controlled.
The goal of this master thesis is then to investigate the potential of metamaterial technology applied to the rotating parts of wind turbines to control and preserve their dynamic behaviour. In particular, the student will explore how to exploit the modulation effect given by the inertial forces to tune the metamaterial system to reduce critical dynamic conditions. Analytical and numerical models will be developed by the student to study the physics and to assess the metamaterial performance applied to such systems. The aim is to propose design guidelines to achieve a robust design solution.
This thesis is in collaboration with Phononic Vibes Srl and is mainly simulation-based.
Suggested Competencies: Python/MATLAB
Advanced Data-Driven Methods for Infrastructure Monitoring and Maintenance Optimization
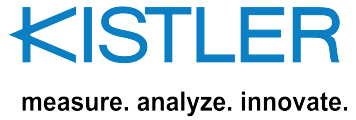
Supervisors: Dr. Charikleia Stoura, Prof. Dr. Eleni Chatzi
Industry Supervisor: Dr. Giulia Aguzzi, KISTLER Instrumente AG
Content
Effective monitoring of civil infrastructure is vital for ensuring long-term safety, serviceability, and cost-efficiency. In recent years, the proliferation of multi-sensor systems and the availability of high-fidelity vibration, acceleration, and Weigh-In-Motion (WIM) data have opened new avenues for developing intelligent monitoring frameworks. These advancements present an opportunity to transition from periodic inspections to predictive maintenance regimes, enhancing asset management strategies in transport infrastructure.
This Master’s thesis aims to develop and benchmark modern AI-assisted methodologies for the analysis of real-world monitoring datasets from bridges and rail infrastructure, with data access supported by KISTLER. The work will focus on synthesizing information from various sources, including vibration signals, tilt measurements, and WIM data, to derive actionable insights into the condition and behavior of structures under operational loading.
The scope includes:
- Developing pipelines for data fusion and pre-processing of multi-sensor time series.
- Performing comparative analyses of existing Structural Health Monitoring (SHM) methods and fault detection strategies.
- Evaluating the added value of WIM data in augmenting structural response interpretation and load estimation.
- Exploring machine learning and signal decomposition techniques (e.g., modal analysis, dictionary learning, supervised/unsupervised ML) for enhanced pattern recognition.
- Investigating tilt estimation through acceleration measurements, with potential use in low-cost sensor deployment scenarios.
- Applying insights to optimize rail surface monitoring and recommend maintenance strategies.
This interdisciplinary thesis connects experimental data analysis, system identification, and data-driven modeling with strong industrial relevance. Students will have the opportunity to work with cutting-edge sensing technologies and contribute toward the digital transformation of infrastructure maintenance.
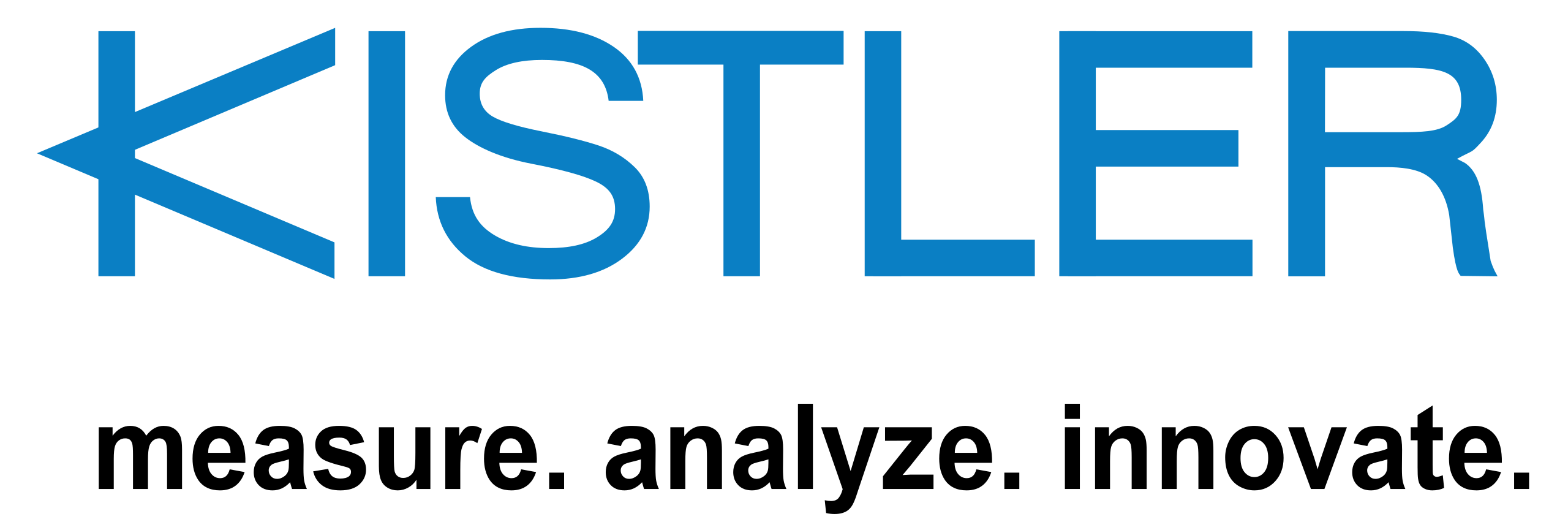
Numerical modelling of a seismically retrofitted building

Supervisors: Dr. Kyriakos Chondrogiannis (), Prof. Dr. Eleni Chatzi
Content
This work will build upon an innovative approach on attenuating seismically induced vibrations on buildings, which is developed at the Chair of Structural Mechanics and Monitoring, ETH Zurich. The objective of this thesis is to model in detail an actual reinforced concrete building that requires upgrades of its seismic performance and study its dynamic behaviour under the suggested retrofit. This work will be part of a larger initiative with the scope to engineer a device (termed NegSV) that can be used in realistic applications. The envisioned device will consist of mechanical components, exploring the concept of negative stiffness. At this stage the modelling of the NegSV will be performed in a simplified manner, while the building structure needs to be modelled in great detail.
Expected project outcomes
- Digitalization/translation of building data from drawings to a Finite Element (FE) model
- Assignment of suitable material properties
- Modelling of the protective device (NegSV) in a simplified manner
- Dynamic analysis of the system under earthquake excitation
- Optimization of the NegSV for efficient vibration attenuation
Required Competencies
Structural mechanics, Structural dynamics, Finite Element Modelling, CAD modelling
Computer programming experience will be beneficial
Software Knowledge
- Proficient in Abaqus or OpenSees
- Autocad/Solidworks or equivalent CAD software. Experience with AutoLISP will be preferable.
- Knowledge of Matlab or Python will be beneficial

Your selection has been recorded
We will follow up with you asap, in order to confirm availability of the selected topic.