Modeling of Nonlinear Monopile Dynamics
new paper out by Tom Simpson
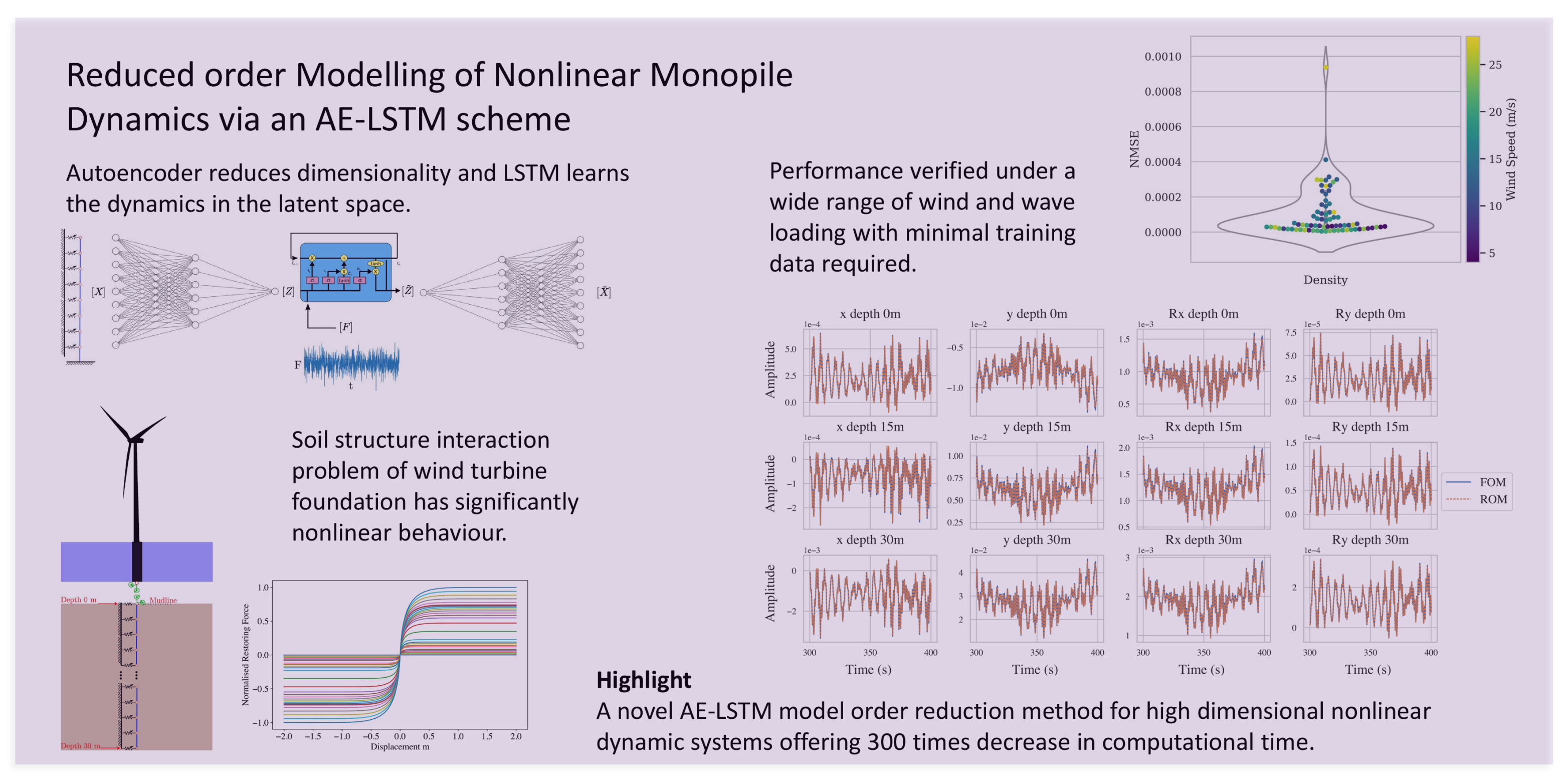
In this work, led by Thomas Simpson (SMM group), with Nikolaos Dervilis (University of Sheffield), Philippe Couturier (Siemens Gamesa), Nico Maljaars (Siemens Gamesa) and Eleni Chatzi (SMM Group), we demonstrate application of a model order reduction strategy exploiting autoencoder and LSTM neural networks (AE-LSTM ROM), for recreating the behaviour of high dimensional nonlinear systems at significantly reduced computational cost. The method requires no prior information on the modelled system and operates exclusively on availability of input output simulations.
Autoencoder (AE) neural networks allow for the reduction of the dimensionality of nonlinear systems and long short term memory (LSTM) networks are then used to learn the dynamics of the modelled system within this reduced representation. By training such a AE-LSTM ROM with a limited amount of training data, we show that the behaviour of a complex nonlinear system, a soil structure interaction (SSI) problem from offshore wind engineering, can be very accurately recreated for different inputs and with a time saving of over 300 times compared to the full order system. Further, the robustness of the method is improved by demonstrating that if the AE-LSTM ROM fails for a certain input time series, that this failure can also be detected.
This project has received funding from the European Union’s Horizon 2020 research and innovation programme under the Marie Skłodowska-Curie grant agreement No 764547 and from the EPSRC under grant agreement EP/R004900/1.
Read more in external page Frontiers in Wind Energy