A Neural EKF for SHM
new article in SHM
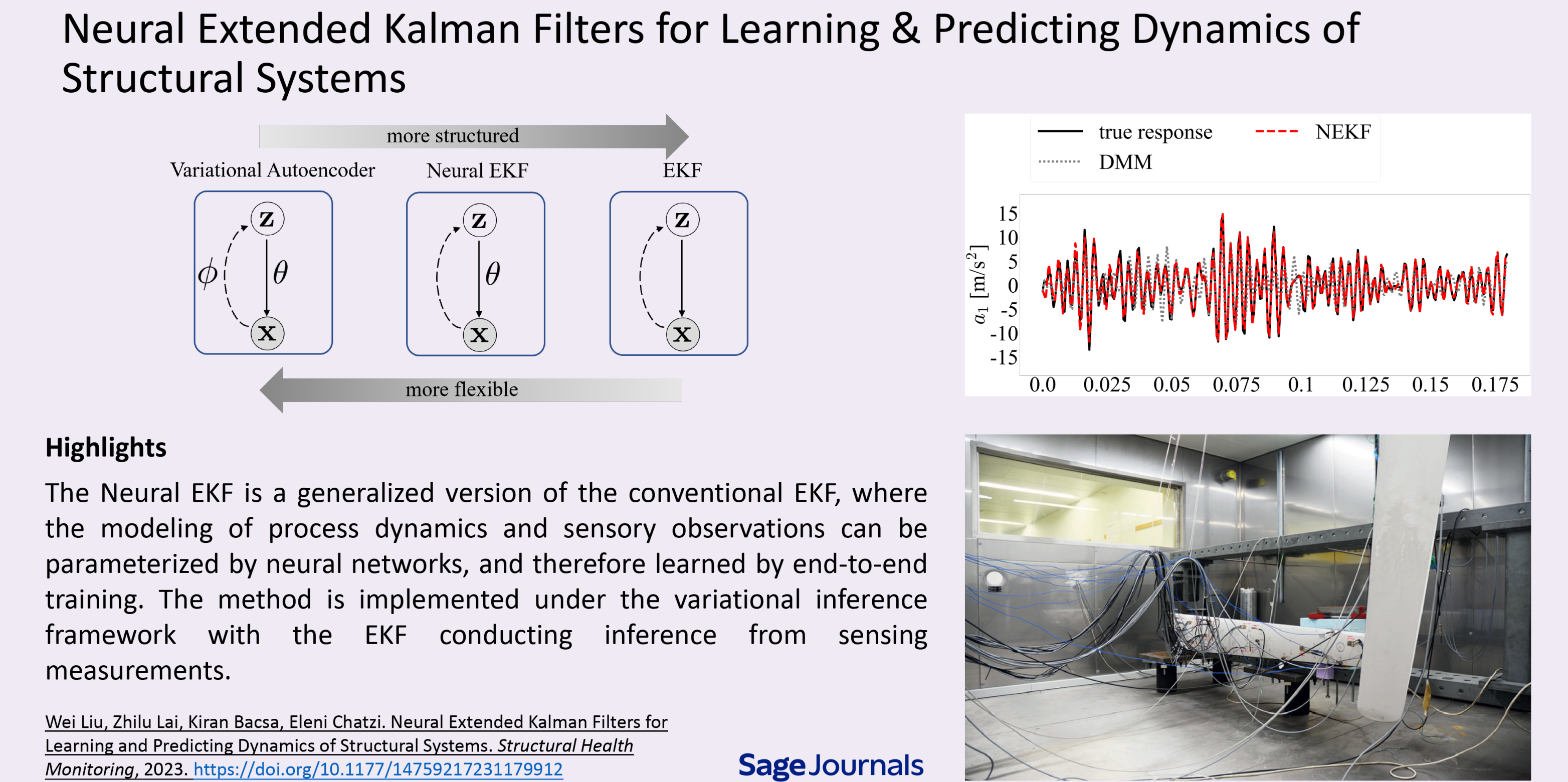
In this work, led by Wei Liu (SEC / NUS), with Zhilu Lai (HKUST), Kiran Bacsa (SEC / SMM group), and Eleni Chatzi (SMM group), we introduce a Neural Extended Kalman Filter (Neural EKF) as a novel approach for accurate structural response prediction in structural dynamics and health monitoring applications. The Neural EKF is a learnable version of the Extended Kalman Filter (EKF), where the modeling of process dynamics and sensory observations are parameterized by neural networks and learned through end-to-end training.
The paper further discusses the limitations of VAE-based methods and conventional Bayesian filtering methods. VAE-based methods often prioritize the inference model over the dynamics model, leading to inaccurate predictions. On the other hand, conventional Bayesian filtering schemes largely rely on known dynamics models, which can be challenging to formulate in scenarios with complex or poorly understood systems. To address these limitations, the Neural EKF combines the advantages of VAEs and Kalman filtering. It leverages the closed-form inference capabilities of the EKF, allowing the training to focus on the dynamics models. Additionally, it eliminates the need for explicitly known transition and observation models required by traditional Bayesian filtering approaches. The Neural EKF is a learnable implementation of the EKF, where the transition and observation models can be learned through end-to-end training, with EKF serving as an effective inference model within the variational learning framework.
The paper demonstrates the framework's value in learning complex dynamical systems through various scenarios, including real-world datasets for seismic response prediction and wind turbine monitoring. The results validate the framework's accuracy in predicting the behavior of physical systems, making it suitable for downstream applications like structural health monitoring and decision making.
This is a collaborative work between the Future Resilient Systems lab at the Singapore-ETH Centre (SEC) and the SMM group.
Read more on external page Structural Health Monitoring.