Bridging POMDPs and Bayesian Decision Making
New paper out by Giacomo Arcieri
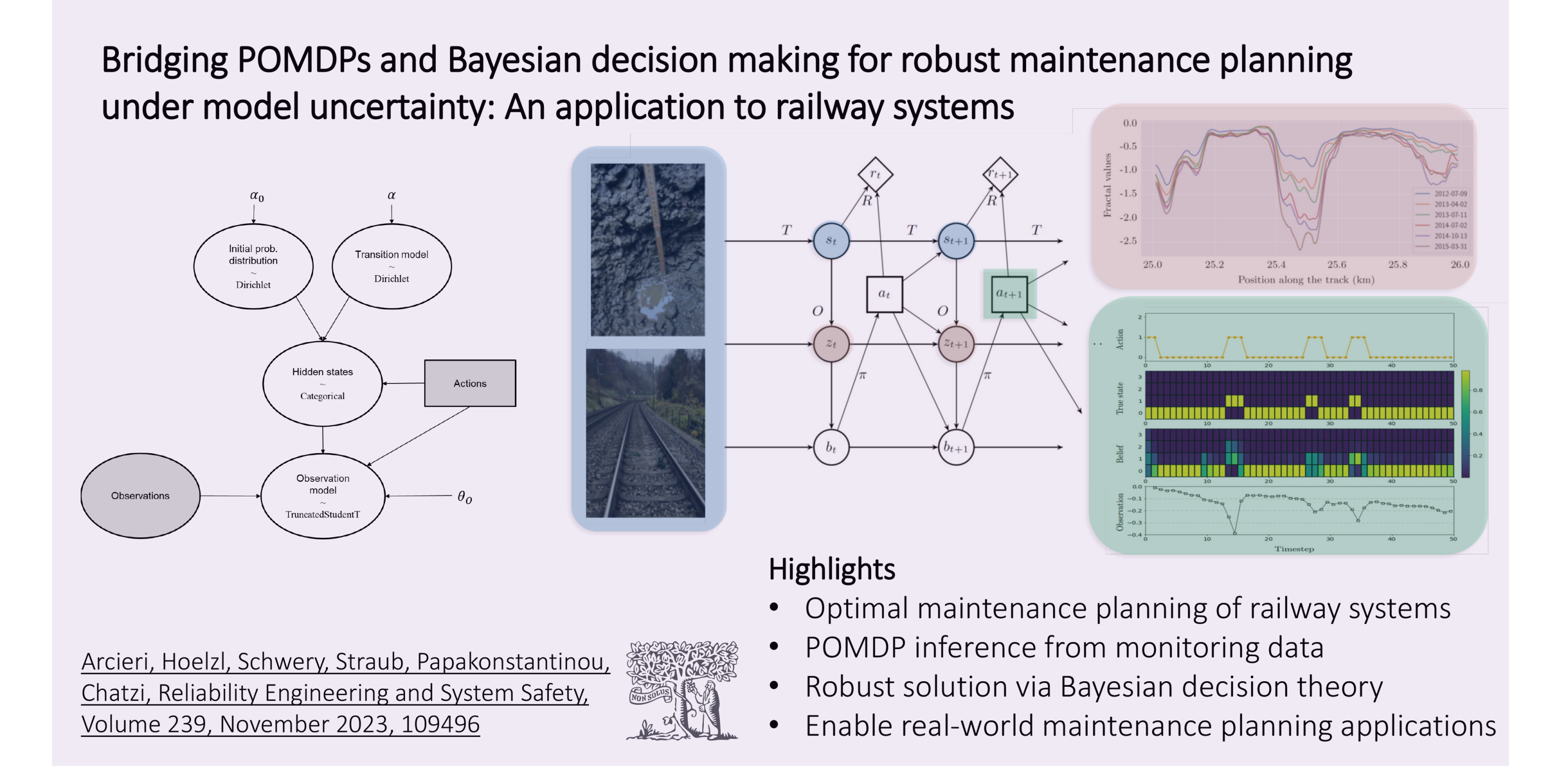
In this work, led by Giacomo Arcieri (SMM Group), we model and solve a real-world optimal maintenance planning of railway assets based on monitoring data as a Partially Observable Markov Decision Process (POMDP). We propose a combined framework for POMDP inference and robust solution built upon real-world data. First, the transition and the observation model parameters are jointly and directly inferred from available data via MCMC sampling (NUTS) of a Hidden Markov Model (HMM) conditioned on actions. The inferred parameter distributions are then incorporated into the POMDP solutions merged with Bayesian decision theory principles to enhance their robustness over model uncertainty. As such, our framework can be used as decision support for planning maintenance actions in deteriorating systems, and considers real-world challenges, such as uncertainty in the observations and in the dynamics of the problem.
If interested in the implementation of the framework, check out our tutorial that demonstrates the step-by-step solution procedure on a simple example, available on external page GitHub.
The work forms a collaboration between the SMM group (Giacomo Arcieri, Cyprien Hoelzl and Eleni Chatzi), our partners at the Swiss Federal Railways - SBB (Oliver Schwery), as well as Daniel Straub (TUM), and Konstantinos Papakonstantinou (Penn State). We further acknowledge the financial support of the Swiss Federal Railways (SBB) as part of the ETH Mobility Initiative project REASSESS.
read more in external page Volume 239 of RESS