SMM at the ICASP 14 Conference
presentation by Giacomo Arcieri
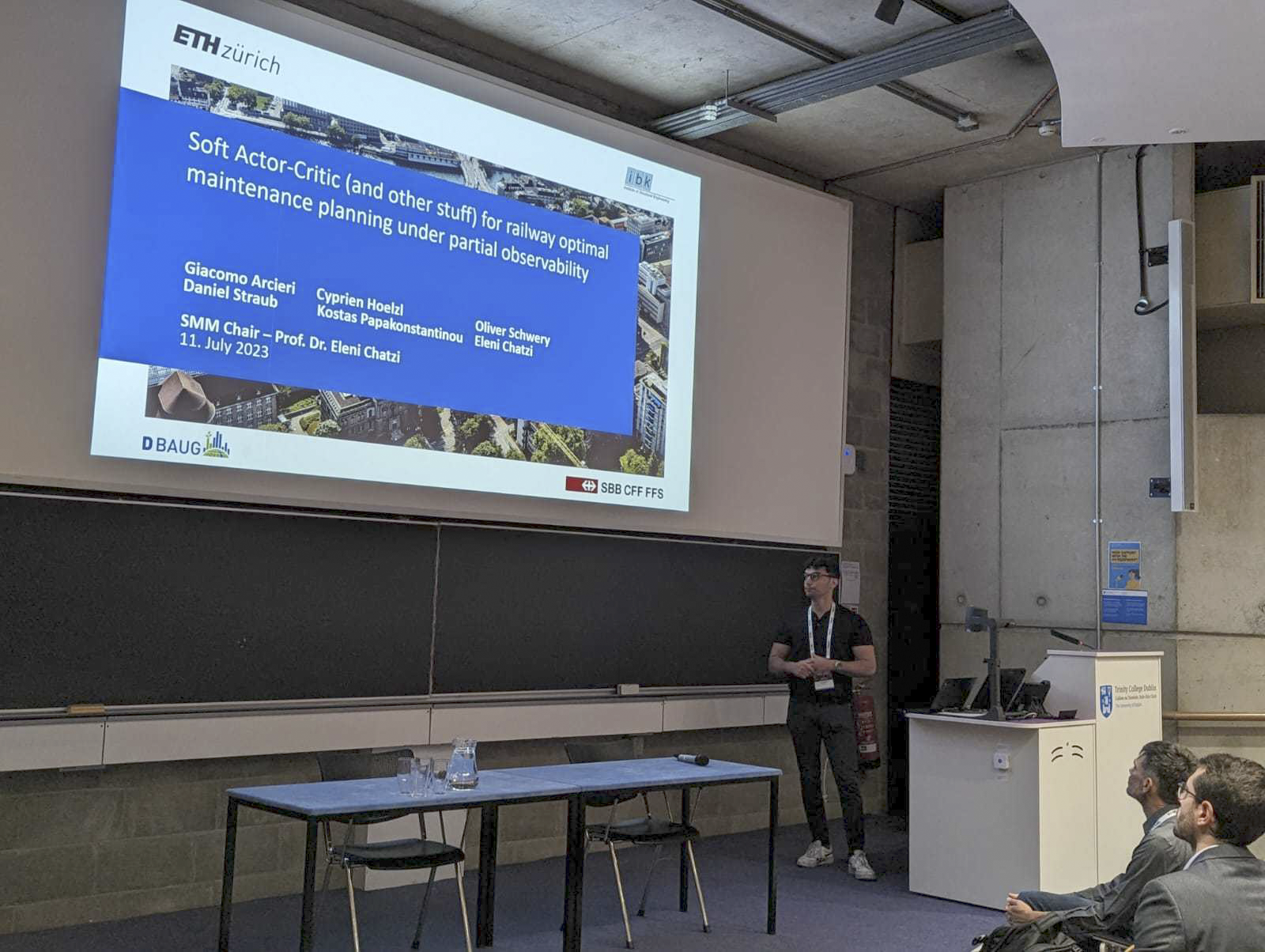
Giacomo Arcieri presented his work on Soft Actor-Critic (and other stuff) for railway optimal maintenance planning under partial observability, during the external page 14th International Conference on Application of Statistics and Probability in Civil Engineering (ICASP 14). The conference took place on July 9th-13th 2023 at Trinity College, Dublin.
His presentation examines the optimal maintenance planning in railway systems as a complex sequential decision-making problem. Optimal maintenance actions ought to be configured on the basis of updated rail condition estimates. To this end, structural health monitoring solutions can be used for reliably tracking the condition of railway infrastructure. However, the measurements gathered from continuous monitoring can only offer incomplete, often noise-corrupted, information of the real condition states, which implies the need for decision–making under uncertainty. For tackling the inherent uncertainty, the problem can be formalized as a Partially Observable Markov Decision Process (POMDP). Two families of methods are generally used to solve such formulations. Dynamic Programming (DP), often also called “planning”, solution techniques can solve small to medium-scale problems with mathematically sound optimality properties. However, they require the full knowledge of the POMDP problem parameters, which govern the deterioration and the repairing effects of maintenance actions, and must thus be known a priori or inferred. On the other hand, Reinforcement Learning (RL) lacks similar convergence properties, but has been empirically proven capable of solving complex tasks of high-dimensionality without prior explicit knowledge of the full set of problem parameters. In this work, we apply a deep RL technique, namely the Soft Actor-Critic method, to solve a real-world railway maintenance planning problem modeled as a POMDP, and compare the quality of the solution against classical DP methods analyzed in previous works.
This work forms a collaboration with Cyprien Hoelzl (SMM Group), Oliver Schwery (SBB), Daniel Straub (TUM), Konstantinos Papakonstantinou (Penn State), Eleni Chatzi (SMM Group).