Data Driven Bridge Influence Lines
in new publication led by Xudong Jian
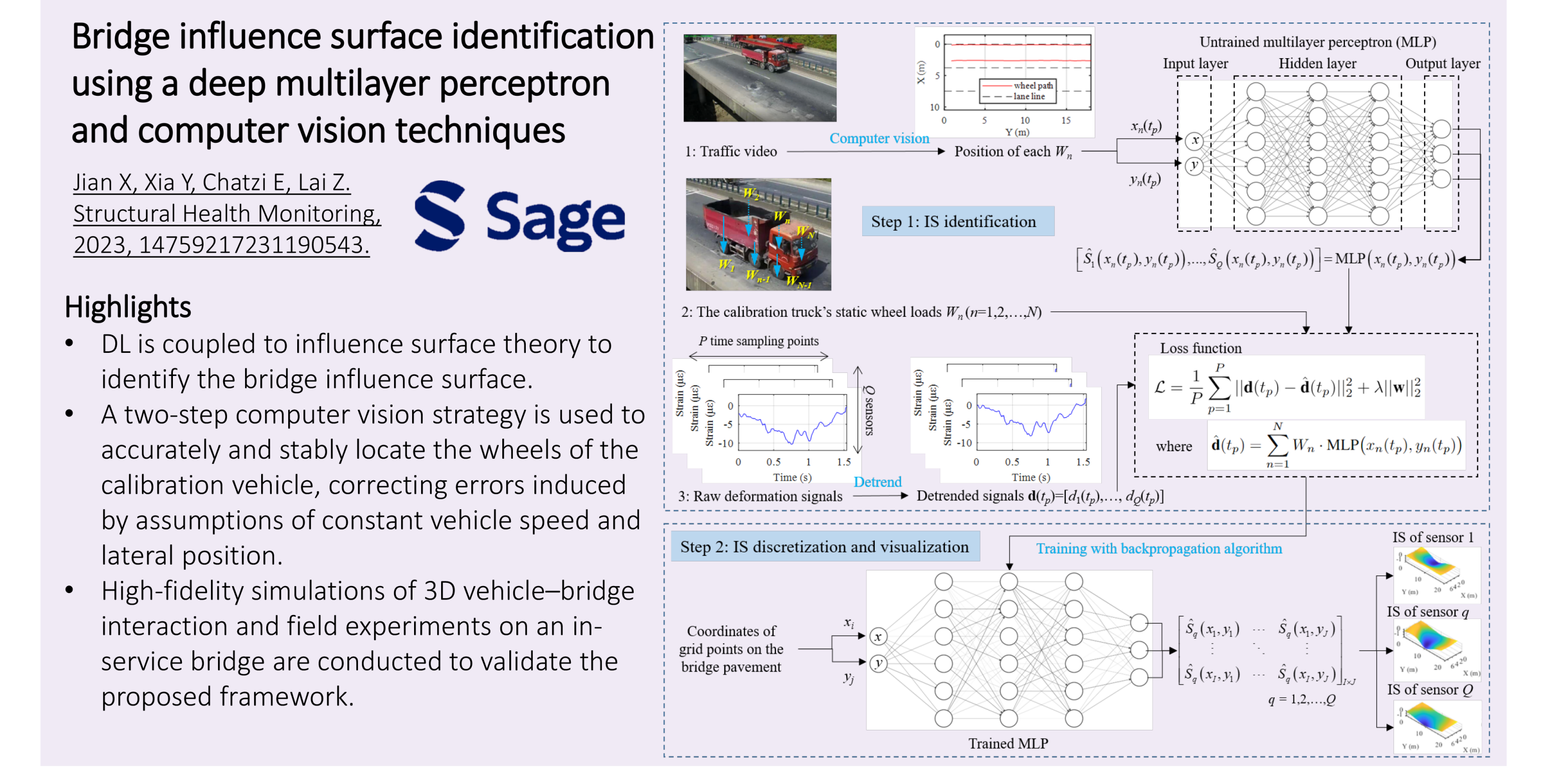
In this work, we propose a deep learning-based method that merges deep multilayer perceptrons (MLPs) and computer vision (CV). We adopt an MLP to identify bridge influence surfaces and structure the training loss function on the basis of the influence surface theory, thus removing the need of simplifying wheel loads as axle loads. By utilizing CV techniques, we are able to precisely locate wheels of the calibration vehicle to reduce errors caused by conventional assumptions of constant vehicle speed and lateral position.
A series of numerical simulations and field experiments on an in-service bridge were carried out to validate the proposed framework and compare it against a broadly established approach to such an end - Quilligan’s method. The results demonstrate the accuracy, robustness, and actionable nature of the proposed framework. If interested in the implementation of our method, check out our codes that are available on external page GitHub.
This work forms a collaboration between the SMM group (Eleni Chatzi, Zhilu Lai) and the Research Division on Structural Health Monitoring and Vibration Control at Tongji University (Ye Xia, Xudong Jian). We further acknowledge the financial support of the National Research Foundation, Prime Minister’s Office, Singapore under its Campus for Research Excellence and Technological Enterprise (CREATE) program, as part of the Future Resilient Systems (FRS) programme.
Read more in external page Structural Health Monitoring - An International Journal (SAGE)