New publication by Weil Liu: Innovative Input Estimation Framework Published in MSSP
Our group at the Singapore-ETH Centre (SEC) and the SMM Group at ETH Zurich presents a novel framework for input estimation in monitored systems using Imitation Learning from Observation (ILfO). Published in MSSP, the method reformulates input estimation as a Partially Observable Markov Decision Process (POMDP), integrating both known and learned dynamics. Validated through simulations and real-world experiments, it marks a significant step in advancing structural monitoring.
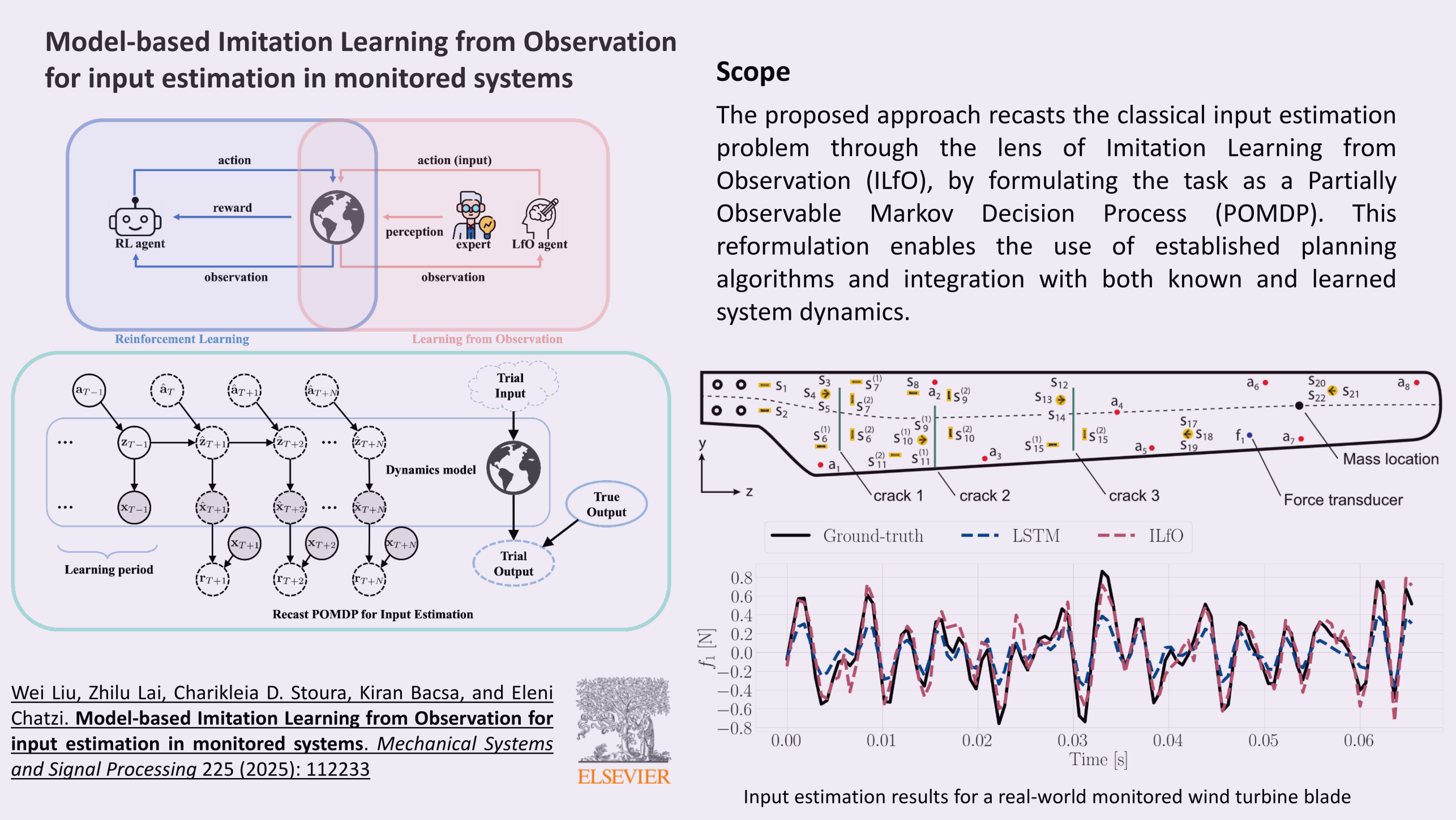
We are pleased to announce a new publication in Mechanical Systems and Signal Processing by researchers from the Singapore-ETH Centre (SEC), the SMM Group at ETH Zurich, and collaborating institutions. This work introduces an innovative methodology for the input estimation problem in monitored systems using Imitation Learning from Observation (ILfO).
Highlights of the Research
- Novel Framework: The study reframes the input estimation problem as a Partially Observable Markov Decision Process (POMDP), enabling the application of established planning algorithms such as the cross-entropy method to achieve robust solutions.
- Integrated Approach: Unlike traditional techniques, this method allows seamless integration with both known and learned system dynamics, enhancing its applicability across a variety of real-world scenarios.
- Validation and Application: The proposed framework was validated on
- A simulated case studies with well-defined dynamics.
- A real-world scaled wind turbine blade experiment, where system dynamics were learned using the Neural Extended Kalman Filter method, demonstrating the method’s robustness in handling imprecisely known dynamics.
This work was led by Wei Liu (Singapore-ETH Centre / National University of Singapore), in collaboration with Zhilu Lai (Hong Kong University of Science and Technology), Charikleia D. Stoura (SMM Group, ETH Zurich), Kiran Bacsa (SEC / SMM Group), and Prof. Eleni Chatzi (SMM Group, ETH Zurich).
Significance
This research represents a significant step forward in leveraging machine learning and system dynamics for input estimation in monitored systems. By adopting a physics-informed learning approach, the methodology contributes to enhancing resilience and predictive accuracy in structural monitoring applications.
📖 Read the full article here: external page https://www.sciencedirect.com/science/article/pii/S0888327024011324