Advances in Multimodal Adaptation and Generalization: A Comprehensive Survey
This new survey paper, led by Hao Dong, explores the evolving landscape of multimodal adaptation and generalization, bridging traditional techniques with the power of foundation models.
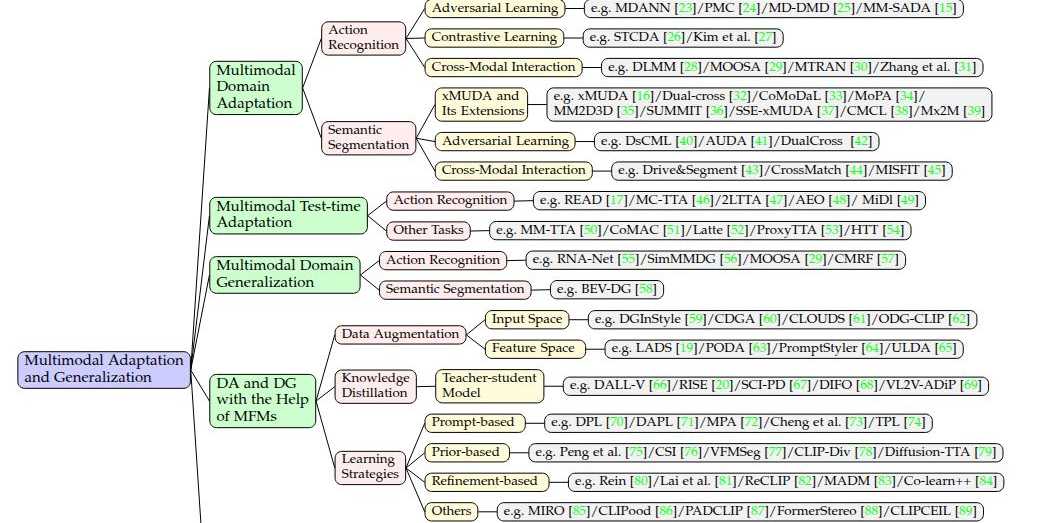
We are excited to announce the release of our latest survey paper, "Advances in Multimodal Adaptation and Generalization: From Traditional Approaches to Foundation Models." This work delves into how AI models can better generalize under real-world distribution shifts, particularly when dealing with multimodal data.
While domain adaptation (DA) and domain generalization (DG) have been well-explored for unimodal data, real-world applications—such as autonomous driving, video understanding, and robotics—require models to effectively handle multiple modalities, including vision, LiDAR, text, and audio.
Our survey provides a comprehensive overview of multimodal adaptation and generalization strategies, from traditional methods to the latest multimodal foundation models (MFMs) like CLIP, SAM, and Diffusion Models.
Key Topics Covered:
- Multimodal Domain Adaptation (MMDA): How to adapt multimodal models across different domains when both source and target data are available?
- Multimodal Test-Time Adaptation (MMTTA): Can multimodal models adapt in real-time without access to source data?
- Multimodal Domain Generalization (MMDG): How can multimodal models be made robust to unseen domains?
- The Role of Multimodal Foundation Models in DA & DG: How can large-scale pre-trained models enhance unimodal adaptation and generalization?
- Adapting Multimodal Foundation Models: Strategies such as prompt tuning and adapters for tailoring MFMs to specific downstream tasks.
📖 Read the full paper: external page Link to paper
📚 Explore our GitHub resources: external page GitHub repository
We welcome discussions and feedback on our findings!
This work is led by Hao Dong, members of the Chair of Strucural Mechanics and Monitoring (led by Eleni Chatzi at ETH Zurich) and the IMOAS Lab (led by Olga Fink at EPFL). We further are thankful for the input by our collaborators: Moru Liu, Kaiyang Zhou, Juho Kannala, and Cyrill Stachniss. The work is supported under the ETH Mobility Initiative coordinated by the Centre for Sustainable Future Mobility - ETH Zurich.