Physics-Encoded GNNs for Resilience Assessment
A new hybrid modelling approach combining GNNs and physics-based ROMs offers a scalable solution for post-earthquake infrastructure assessment , demonstrate on the case study of Interconnected Electrical Equipment components.
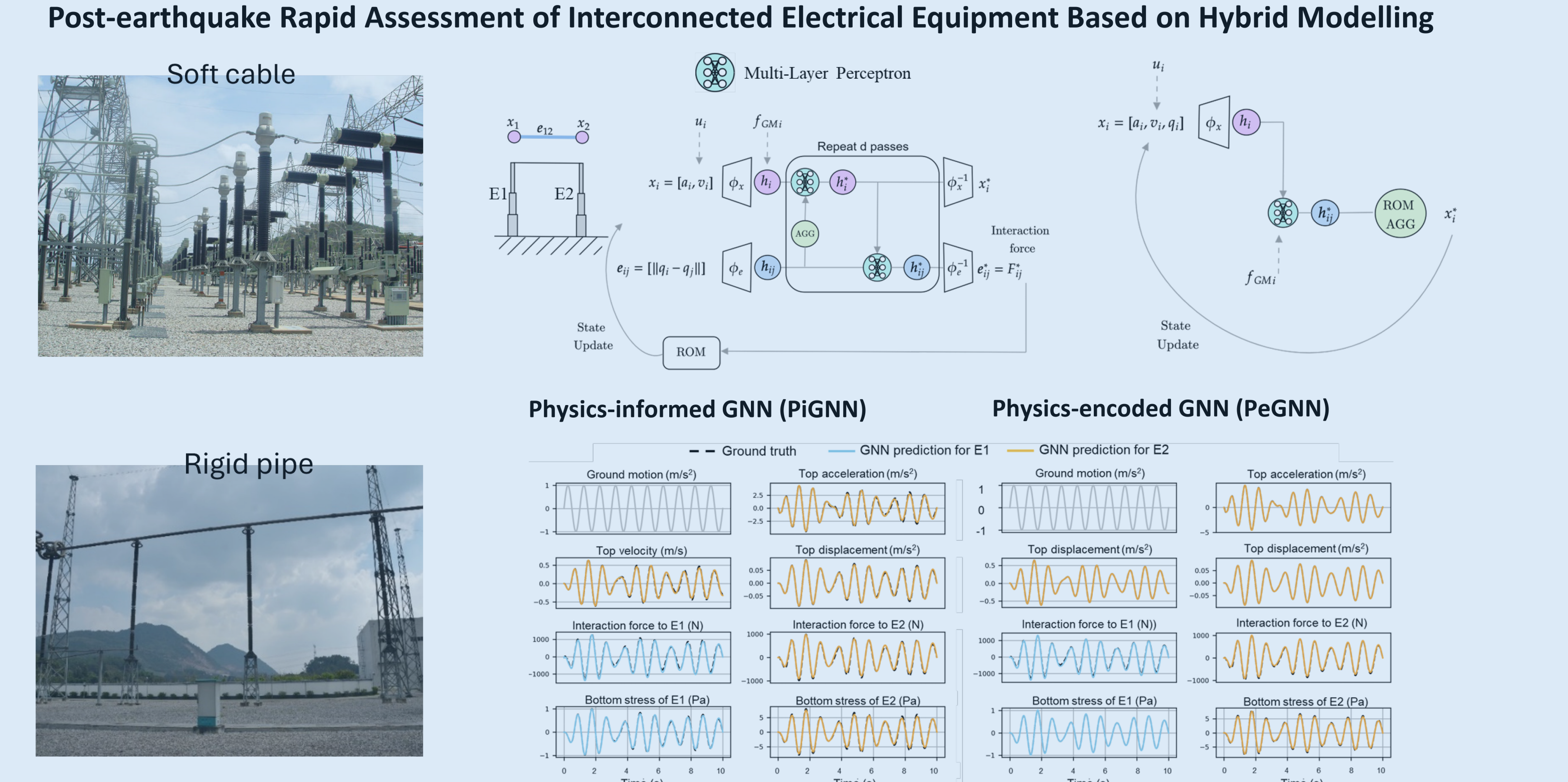
A recent publication from the 10th Asia-Pacific Workshop on Structural Health Monitoring (APWSHM) showcases a novel methodology developed by an international team of researchers, including members of the SMM group and Future Resilient Systems (FRS) at ETH Zurich.
The work, led by Beatriz Moya (ENSAM) and Huangbin Liang (FRS and SMM Chair), in collaboration with Francisco Chinesta (CNRS and CNRS@CREATE) and Eleni Chatzi (FRS and SMM Chair, ETH Zürich), introduces a hybrid modelling framework for the seismic assessment of interconnected electrical infrastructure.
The proposed method integrates Graph Neural Networks (GNNs) with physics-based reduced-order models (ROMs) of standalone components, enabling the inference of interaction forces and the prediction of system-wide dynamic behavior, all while minimizing the need for extensive sensor networks.
Key advantages of this approach include:
- Capturing spatial interactions via graph representations
- Modeling temporal evolution through recurrent computations
- Enabling rapid, post-earthquake condition assessments
- Supporting emergency repair planning through interpretable results
The approach is validated using a case study involving 800 kV post insulators interconnected by busbars, demonstrating its potential to inform cost-effective and accurate resilience assessments for critical power infrastructure.
📖 The full paper is available as part of the external page APWSHM 2024 proceedings