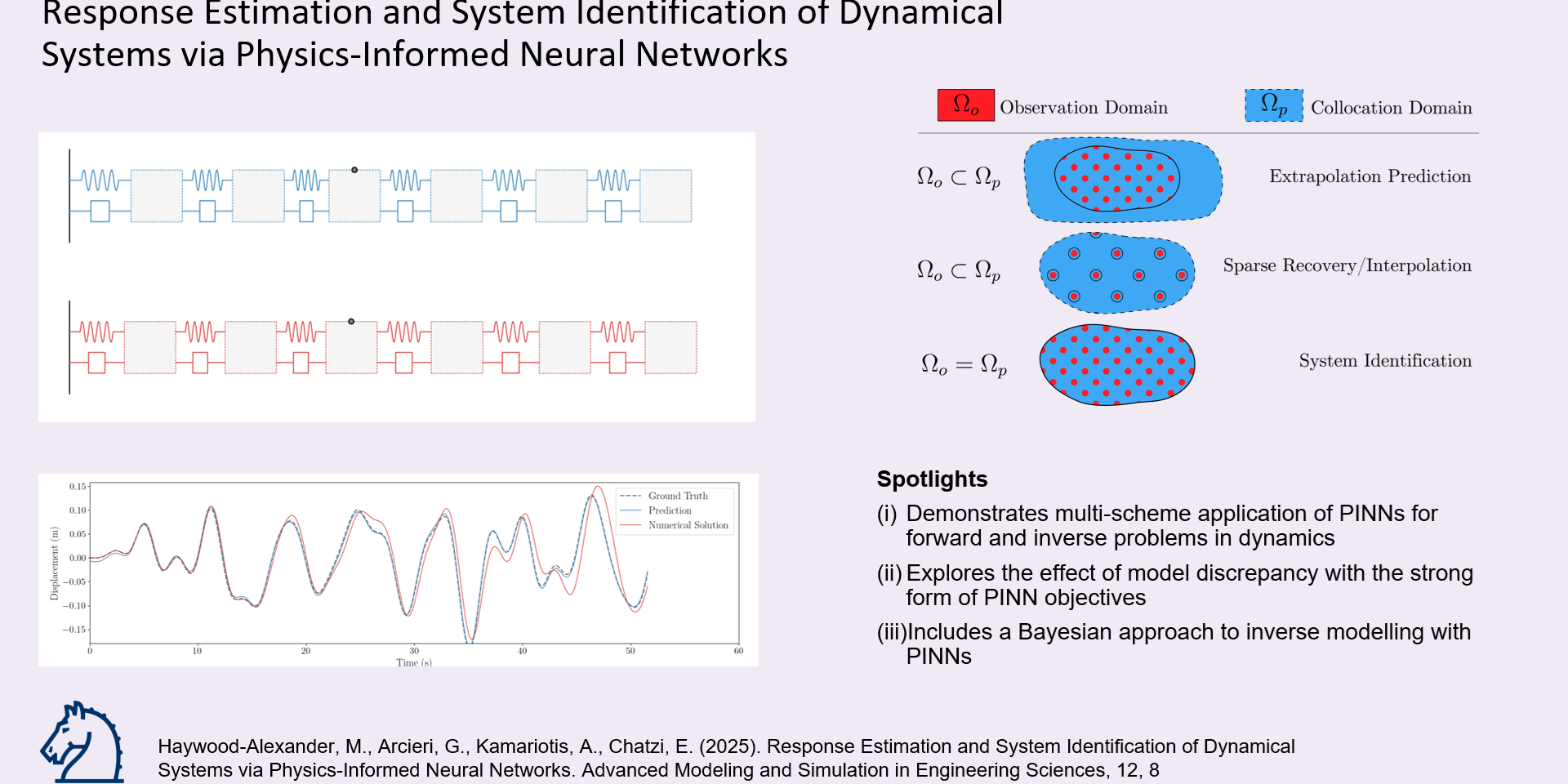
Physics-Informed Neural Networks for Dynamical System Identification
A recent study led by Marcus Haywood-Alexander, in collaboration with members of the Chair of Structural Mechanics and Monitoring, explores the potential and limitations of Physics-Informed Neural Networks (PINNs) for system identification in dynamical settings.
We are pleased to share the latest publication from our group:
“Response estimation and system identification of dynamical systems via physics-informed neural networks,” now published in Advanced Modeling and Simulation in Engineering Sciences and available external page open access here.
This work is led by Marcus Haywood-Alexander and co-authored by Giacomo Arcieri, Antonios Kamariotis (now with Hitachi Energy), and Eleni Chatzi. It investigates the application of PINNs to both forward and inverse problems in structural dynamics, with a particular focus on estimating state variables, system parameters, and their joint identification.
Beyond showcasing the promise of PINNs for response estimation under sparse measurement conditions, the study takes a critical look at their behavior under model uncertainty. It addresses the following key questions:
How do PINNs perform when the assumed model deviates from reality?
What is the effect of incorporating model discrepancy within strong-form PINN formulations?
How can Bayesian approaches complement PINNs for robust inverse modelling?
The work contributes to the growing field of Physics-Enhanced Machine Learning (PEML), offering valuable insights into the opportunities and boundaries of integrating physical knowledge with data-driven methods.
We welcome engagement from the community and look forward to future discussions and collaborations in this exciting research area.